Five Cognitive Biases That Can Skew Your A/B Testing Decisions
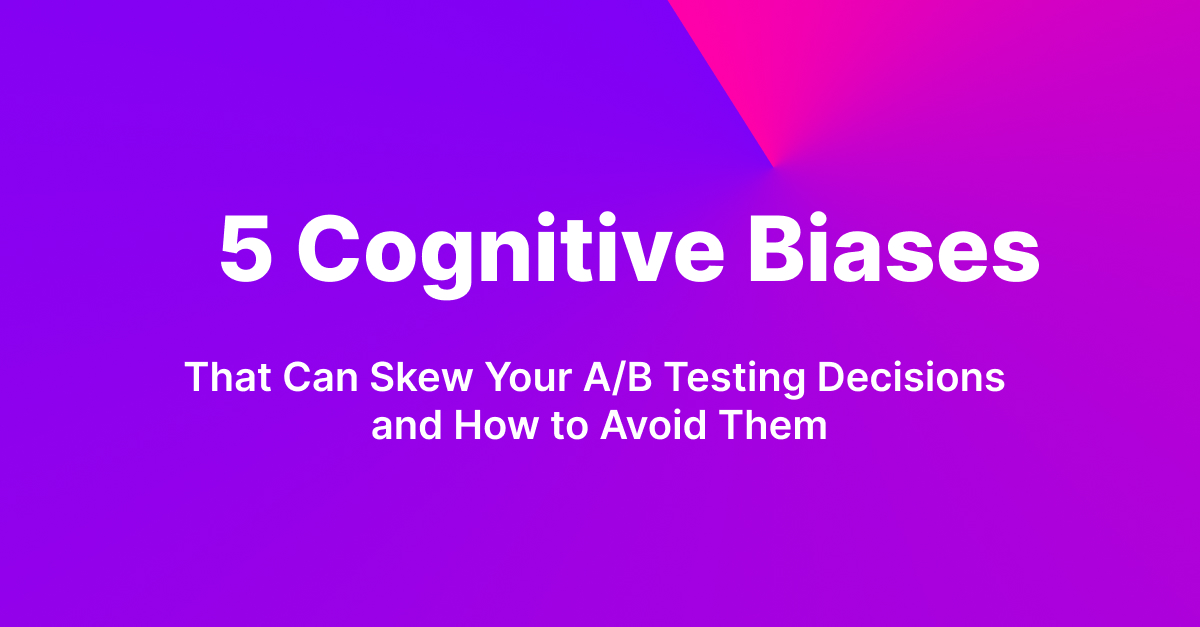
Introduction
Even the most data-driven among us are human at heart – and humans come with cognitive biases. Cognitive biases are mental shortcuts or tendencies that subconsciously influence how we interpret information and make decisions. In A/B testing and conversion rate optimization (CRO), these biases matter because they can cloud our objectivity. If you’re not careful, biases can creep into your test hypotheses, how you pick winners, and how you interpret results. In other words, a biased mindset can lead to misleading test results or wasted opportunities – you might see what you want to see in the data instead of what’s really happening. As Speero’s experimentation experts put it, our cognitive biases can even make us dismiss new ideas that challenge our beliefs. That’s a recipe for missing out on real insights.
The first step is awareness. Let’s shine a light on five key cognitive biases that frequently impact marketers and CRO specialists when setting up or analyzing A/B tests. We’ll explore each bias in a conversational way (with a dose of relatable examples) and then discuss how to mitigate these biases so your experiments stay as objective and evidence-based as possible.
1. Confirmation Bias
Have you ever run a test hoping for a certain outcome, and then found yourself cherry-picking the data that supports your hypothesis? That’s confirmation bias in action. By definition, confirmation bias is our tendency to favor information that confirms our assumptions or preconceptions. In practice, a marketer might latch onto metrics or anecdotes that validate their hunch, while overlooking evidence that contradicts it.
In A/B testing, confirmation bias can lead you to design experiments in a one-sided way or interpret results with rose-colored glasses. For example, say you believe a flashy new homepage will outperform the old one. If early data shows a slight uptick, you might immediately celebrate and ignore later data that suggests the lift wasn’t significant. As one CRO author notes, confirmation bias often involves “long-held beliefs and our unwillingness to admit we’re wrong”. We might even set up a hypothesis like “Version A will definitely beat Version B because it aligns with previous trends,” which doesn’t allow room for the possibility we could be wrong.
Why it’s problematic: Confirmation bias means we’re not truly testing – we’re simply seeking validation. This can result in false positives (seeing a “winner” where none exists) or missed learnings. If we ignore evidence that a variation isn’t actually better, we might implement a change that doesn’t move the needle (or could even hurt conversions in the long run).
Real-world check: To catch yourself, ask: “Am I objectively looking at all the data, or just the parts that support my story?” A healthy test culture treats a surprising result as a learning, not an inconvenience. Remember, an A/B test that proves you wrong isn’t a failure – it’s valuable new insight about your audience.
2. Survivorship Bias
Marketers love success stories – blog posts and case studies where a simple tweak led to a 300% conversion lift. It’s inspiring, but it can also be deceptive. Survivorship bias is the tendency to focus on the “survivors” (the winners) and ignore the “casualties” or failures that we don’t see. In A/B testing, this often means we pay undue attention to successful tests and gloss over the many tests that showed no lift or a negative result.
Why is this a problem? Because it skews our expectations and decision-making. If you only look at winning case studies from others, you might assume every test you run will be a home run – which is far from reality. In truth, industry data shows most tests are inconclusive or produce only minor improvements. Companies rarely publish case studies about experiments that failed or produced no significant change. As one marketing blog quips, “Not every A/B test is successful, or will produce meaningful results. Companies that run failed A/B tests typically do not write case studies about this failure… Case studies only show outliers — the most remarkable stories of success… when you don’t hear about negative outcomes, you are looking at a biased sample.”
Survivorship bias can also occur internally. Let’s say your team ran 10 tests last quarter, but only remembers the two big wins. If you base your strategy only on those, you might misattribute what truly caused the wins or repeat tactics that don’t actually work consistently. You’ve “survived” only the winning data points.
How to avoid it: Be sure to study the losers and the inconclusive tests, not just the winners. There’s often as much (or more) to learn from a test that failed to beat the control. Maybe your new landing page didn’t win because the messaging missed the mark – that’s useful knowledge for future hypotheses. When looking at others’ case studies, keep a skeptical eye and remember you’re seeing a highlight reel. As CRO agencies often remind clients, you need a lot of experiments (including duds) to discover a few big breakthroughs. Don’t let the flashy success stories give you tunnel vision.
3. Anchoring Bias
Anchoring bias occurs when we rely too heavily on the first piece of information we receive (the “anchor”) when making decisions. In A/B testing, anchors pop up all over the place: the first day’s test results, an old benchmark figure, or even a preconceived number we had in mind. Our brain gives undue weight to that initial data point and uses it as a reference for everything that comes after.
Imagine you launch an experiment and by mid-day the new variant is up +20%. That initial spike can become an anchor. You might start expecting a huge lift, and any result smaller than +20% now feels like a letdown – or worse, you might call the test early, declaring victory based on the early returns. Anchoring can also happen if, for example, last month’s conversion rate was 5% and you mentally anchor on 5% as the baseline for any new test. If you see 5.5%, you assume it’s an improvement, even if seasonality or randomness could be at play.
The danger is obvious: acting on incomplete or skewed information. It’s like calling an election winner with only 10% of the votes counted. A marketing tech article used that exact analogy to describe how some teams make the mistake of declaring A/B test winners way too soon. Early results can be misleading – maybe your test got a rush of enthusiastic early adopters, or perhaps there was an error in tracking that gave a false initial read. If you anchor on that, you might stop the test or shift resources prematurely.
Combating anchoring bias: Set predetermined rules for your tests before you see any data. For instance, decide on a minimum test duration and sample size upfront (e.g., “We won’t look at results or pick a winner until the test runs for two full weeks and at least 1,000 conversions per variant”) so that you’re not tempted to be swayed by the first data points. Many CRO specialists also recommend blinding yourself to interim results – some tools allow you to hide performance data until a test is complete. By delaying gratification, you ensure that the “first impression” doesn’t derail your objective analysis. In short, don’t let the first squeak of data shout in your ear louder than the complete dataset.
4. Recency Bias
Recency bias is the flip side of anchoring in a way – instead of overvaluing the first data, we give too much weight to the most recent data. In other words, the latest trend or outcome starts to dominate our thinking, at the expense of historical context. In A/B testing, recency bias might lead you to overreact to a short-term fluctuation or assume that a pattern you saw in the last few tests will continue indefinitely.
For example, suppose your last three experiments all showed a lift from adding urgency messaging (like countdown timers or limited-time offers). It’s easy to develop a belief that “users are currently really motivated by urgency.” Now, that might be true in the short term or for those specific tests. But if you start applying this to everything – say, slapping a countdown timer on every page – without considering the broader picture, you could run into trouble. We tend to overvalue recent performance trends against what we’ve learned from past tests or different environments . The danger is generalizing results across your entire program without checking if the context is comparable.
A classic example: An e-commerce team finds that in the last few weeks, mobile conversion rates have spiked after a site redesign. They might be tempted to attribute this entirely to the redesign and shift all focus to mobile. But if those weeks were also during a holiday sale, the recency bias could fool them – it might have been the seasonal surge driving conversions, not just the design change. As another scenario, an email marketer runs an A/B subject line test right before Mother’s Day and finds an emotional subject line crushed a more generic one. That insight is useful, but recency bias would be assuming “emotional messaging always wins” going forward. Context matters (a last-minute Mother’s Day campaign is different from, say, a back-to-school campaign) and what’s true recently may not hold true later.
How to keep recency bias in check: Always zoom out before you decide. Look at a broader time horizon or multiple data points. If a recent test yielded a surprising result, compare it with historical data – is this a blip or part of a long-term trend? When possible, validate a newfound “trend” by running follow-up tests in different time periods or segments. The key is not to get overconfident about a single recent outcome. As the saying goes, “one data point is a dot, two is a line, three is a trend” – make sure you have enough evidence before reshaping your strategy around the latest thing. Staying grounded in long-term metrics (monthly/quarterly conversion rates, year-over-year comparisons, etc.) can also provide context so you’re not swept up by the moment’s excitement.
5. The False Consensus Effect
Ever heard a colleague say something like, “I just know our users will love this new feature, everyone will use it,” or the opposite, “No one likes [insert element]; I hate it so our customers will too”? This is a prime example of the false consensus effect. It’s our tendency to overestimate how much other people agree with our own beliefs or preferences. In the realm of CRO and UX, we often fall into the trap of thinking our personal opinion represents the majority of users.
In A/B testing, the false consensus effect might influence which ideas you test or how you predict results. If you personally dislike pop-up forms, you might assume any test involving a pop-up will tank because “everyone hates pop-ups.” But is that objectively true? Often, it’s not. As ConversionXL humorously pointed out, marketers commonly say things like “No one clicks on pop-ups” or “Everyone hates sliders,” projecting their own feelings onto the entire user base. In reality, you may find a well-timed pop-up actually converts quite nicely, despite your personal distaste for it. The danger of false consensus is that it can cause bias in your test prioritization – you might never test a bold idea because you assume users won’t like it (when in fact, you never asked them!), or you might interpret results with a heavy personal filter.
This bias also appears in how we interpret qualitative feedback. Say you do a few user interviews or read through some survey responses. If one participant’s opinion aligns with yours, you might give it more weight (“See, I knew most users felt this way!”) than other feedback. We tend to seek consensus with our own viewpoint, sometimes imagining it’s there when it isn’t.
How to avoid falling for false consensus: Embrace data and diversity of opinions. Always remind yourself, “I am not the user.” Your preferences are valid, but they may not reflect your audience at large. When crafting hypotheses, base them on user research, analytics, or observed behavior – not just gut feelings. If you catch yourself making a sweeping statement about “everyone” or “no one,” pause and consider testing that assumption. Many CRO agencies encourage testing even those ideas you think won’t work, because you might be surprised. Also, when gathering feedback, look for patterns across many users, not just the ones who echo your own thoughts. In summary: let your users tell you what they want through actual behavior. Your role is to listen and experiment, not to assume you already know the consensus.
How to Mitigate These Biases in A/B Testing
By now it’s clear that cognitive biases are sneaky – they operate in the background of our minds. The good news is, knowing is half the battle. Once you’re aware of these biases, you can take concrete steps to mitigate them. Here are some best practices from CRO experts to help keep your A/B testing unbiased and on track:
• Acknowledge and Embrace Being Proven Wrong: First, cultivate a mindset where data is the boss. Be okay with the idea that your hypothesis might be wrong. In fact, expect that many will be. This “epistemic humility” (being willing to rethink your beliefs) is crucial for experimentation success. Teams that celebrate learning over ego create a safe space where biases are called out. Simply put – acknowledge your cognitive biases exist, so you stay vigilant about them when planning and analyzing tests.
• Frame Neutral, Testable Hypotheses: When designing tests, write your hypothesis in a neutral way that focuses on discovery, not on confirming your preconceived idea. For example, instead of hypothesizing “Changing our CTA to red will increase conversions because I believe red is more attention-grabbing,” frame it as, “We are testing if changing the CTA color to red versus blue affects conversions.” This way, you’re not mentally stacking the deck in favor of one outcome – you’re genuinely curious about what will happen. A neutral hypothesis keeps you honest and open-minded, reducing confirmation bias from the start.
• Pre-define Your Test Plan (and Stick to It): One of the strongest safeguards against biases like anchoring and primacy is to set your analysis plan upfront. Determine your sample size, test duration, and criteria for significance before you launch the test. For instance, decide that you’ll run the experiment for a full two weeks (or until X conversions) no matter what the early data says. And don’t peek at results halfway through – resist the urge! As a best practice, avoid reviewing results mid-test to prevent the “freak-out factor” that makes someone hit the stop button too soon. By waiting until you have a complete dataset (and statistical significance), you ensure early noise or random fluctuations don’t mislead you. In short, patience and discipline in following the scientific method will save you from many a bias-driven mistake.
• Look at the Full Picture of Data: To combat all sorts of biases, always challenge yourself to consider all relevant metrics and segments, not just the ones that make your idea look good. Maybe Variant A had a higher overall conversion rate, but did it perform uniformly across traffic sources? What if mobile users loved it but desktop users hated it? Digging deeper can reveal if you’re wearing blinders. Also, use both quantitative and qualitative data in tandem. Numbers tell you what happened; qualitative insights (like session recordings or user feedback) can help explain why. By immersing yourself in multiple perspectives (analytics, heatmaps, user surveys, etc.), you’re less likely to latch onto a single narrative that confirms your bias.
• Use Tools and Automation to Reduce Human Bias: Modern experimentation platforms and statistical tools can be allies here. Many A/B testing tools have features to enforce test length or to auto-calculate significance, taking those decisions out of your hands. Some organizations even use AI-powered analysis to interpret results without emotional bias – for example, anomaly detection algorithms that signal if a result is likely noise. Additionally, techniques like sequential testing or false discovery rate controls can minimize false positives, ensuring that what you deem a “win” truly is one. The idea is to lean on objective frameworks. An algorithm isn’t subject to impatience or ego – it won’t stop a test early because it thinks it sees a pattern. While humans must ultimately make the decisions, using these tools can keep us honest. As one expert advises, a structured testing methodology aligned to your goals will help avoid on-the-fly decisions that biases influence.
• Team Reviews and Second Opinions: Don’t run your testing program in a silo. Involve others in brainstorming hypotheses and reviewing results. Colleagues might spot bias in your reasoning that you missed. For instance, do a quick bias check in experiment post-mortems: “Are we anchoring on an outlier? Are we assuming all users behave like us?” A/B testing is a team sport in successful companies – when you foster a culture where team members feel comfortable challenging assumptions, you create natural checks and balances against individual cognitive biases.
By applying these practices, you create an environment where data and evidence trump intuition and biases. It’s about building a culture of objective experimentation – something every CRO agency will tell you is key to sustained success.
Conclusion
Cognitive biases are an inevitable part of human decision-making, but they don’t have to derail your A/B testing efforts. The key takeaways: be aware of these five common biases (confirmation, survivorship, anchoring, recency, and false consensus) and actively guard against them. Treat every experiment as a learning opportunity, whether it confirms your hypothesis or shatters it. Encourage a culture where challenging assumptions is welcome and “I was wrong” is met with high-fives for uncovering the truth.
Remember that the goal of CRO is not to prove yourself right – it’s to find what truly works for your users. As one article noted, no matter how carefully you plan, no testing program is completely immune to bias, but recognizing those biases and sticking to a solid process can minimize their impact and strengthen your confidence in the results . In practice, that means making decisions based on data, not whims, and being willing to pivot when the data tells a different story than expected.
In summary, stay curious and skeptical (in a healthy way). Let the evidence from your users guide you, and use each experiment – win or lose – to iterate towards better user experiences. If you do that, you’ll mitigate the worst of these biases. The result? More reliable A/B test outcomes, more valid insights, and ultimately, higher conversion lifts that aren’t just illusions of our psychology, but real improvements that drive your business forward. Here’s to keeping our experimentation honest and our minds open!
What if there were no biases?
What if optimization decisions weren’t clouded by human biases—no gut-driven changes, no cherry-picked data, no over-reliance on the first “winning” test? AI removes these mental traps by analyzing vast datasets objectively, adapting in real time, and continuously optimizing based on actual user behavior. Instead of costly missteps, AI-driven optimization delivers better outcomes with less effort—because data, not instinct, leads the way.
That’s exactly why we built ezbot. Our AI-powered optimization engine sidesteps human biases, automatically learning what works best for each visitor—without endless A/B tests or guesswork. The result? Higher conversions, better user experiences, and less time spent tweaking. Get started today and let AI do the optimizing for you. 🚀