AI-Powered Testing: The Next Evolution in Optimization

In this guide, we explore how AI is revolutionizing digital experience optimization, moving beyond traditional A/B testing to enable dynamic personalization, predictive modeling, and real-time adaptation. Drawing on ezbot's pioneering work in AI-powered testing, we'll examine the limitations of conventional methods, reveal breakthrough capabilities now possible, and provide a roadmap for implementing these advanced approaches in your organization. Discover how leading companies are achieving dramatic conversion improvements through intelligent, contextual optimization that adapts to each user's unique circumstances.
The Transformation of Digital Experience Optimization
The landscape of digital optimization has undergone a remarkable evolution. What began as simple A/B testing—comparing two webpage variants to see which performs better—has matured into a sophisticated discipline that increasingly leverages artificial intelligence to drive unprecedented results. Today, organizations at the forefront of digital experience optimization are embracing AI not just as a supplementary tool but as a fundamental paradigm shift in how we approach testing and personalization.
This shift represents more than just technological advancement; it signals a fundamental change in how we conceptualize the optimization process itself. Traditional A/B testing methodologies, while valuable, are increasingly recognized as just one component in a more comprehensive, AI-driven approach to understanding and enhancing user experiences. At ezbot, we're at the forefront of bringing these technologies to the masses. Today, we're bringing our knowledge to you.
Beyond Traditional A/B Testing Limitations
Traditional A/B and multivariate testing methodologies have served as the backbone of conversion optimization for nearly two decades. These methodologies provided a structured, scientific approach to improving digital experiences, enabling organizations to make evidence-based decisions rather than relying on intuition alone. However, these conventional approaches have inherent limitations that AI-powered testing is now addressing:
1. Limited Test Velocity and Scope
Traditional testing platforms constrain organizations to testing a handful of variations simultaneously. Even with multivariate testing, the combinatorial explosion of options quickly becomes unmanageable. Teams must carefully prioritize which elements to test, potentially missing valuable optimization opportunities. AI systems can evaluate vastly more combinations simultaneously, identifying winning variations that human testers might never have the bandwidth to discover.
2. Static Visitor Segmentation
Conventional testing platforms rely on predefined segments based on relatively simple criteria. This approach fails to capture the nuanced ways in which different user types might respond to variations. AI can identify complex patterns in user behavior that aren't immediately obvious to human analysts, creating dynamic segments that continuously evolve as new data becomes available.
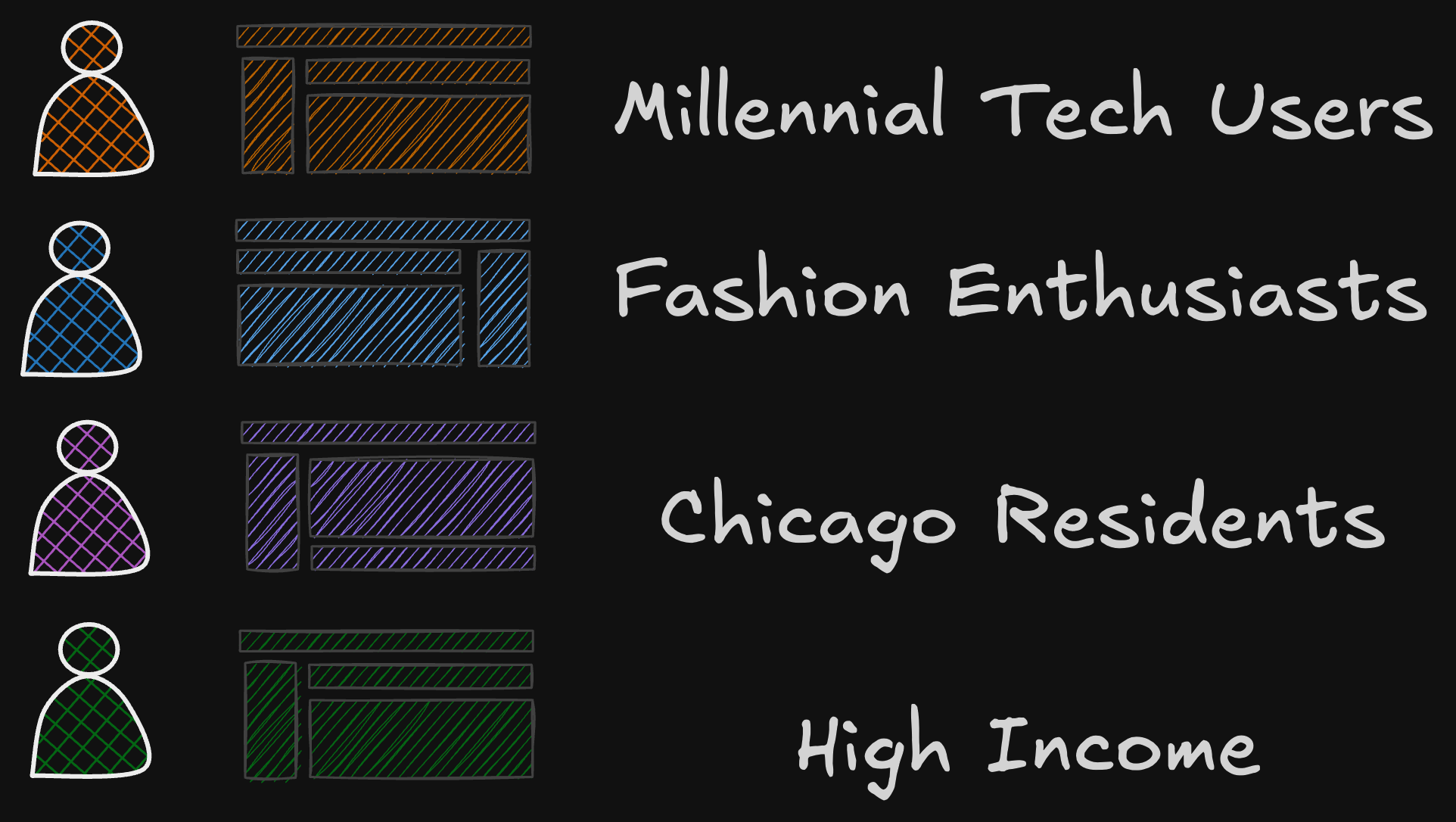
3. Linear, Sequential Testing Processes
Traditional optimization follows a linear pattern: hypothesize, test, analyze, implement, repeat. This methodical approach, while sound, is inherently slow and lacks the agility to respond to rapidly changing user behaviors or market conditions. AI-powered systems can run continuous, parallel experiments, learning and adapting in real-time without requiring manual intervention at each stage.
4. Analysis Bottlenecks
Interpreting test results often becomes a bottleneck in traditional testing programs. Data analysts must manually dig through results, segment by segment, to uncover insights. AI systems can automatically identify patterns across countless dimensions simultaneously, surfacing unexpected insights that might otherwise remain hidden in the data.
Key Components of AI-Powered Testing
The integration of AI into optimization programs introduces several transformative capabilities that extend far beyond what traditional testing methodologies can offer:
Automatic Insight Generation
AI systems continuously analyze vast datasets, identifying patterns and correlations that would be impractical for human analysts to discover manually. These systems can automatically flag underperforming segments, detect anomalies in user behavior, and suggest potential optimization opportunities based on observed patterns.
Predictive Modeling and Simulation
Advanced AI systems can simulate user interactions with different experience variations before implementing them in live environments. These predictive models leverage historical data to forecast how different segments will likely respond to potential changes, allowing teams to narrow their focus to the most promising variations.
Dynamic Traffic Allocation
Unlike traditional A/B tests with fixed traffic splits, AI-powered systems can dynamically adjust traffic allocation in real-time based on performance. These systems (often referred to as "multi-armed bandits") balance exploration (gathering data about different variants) with exploitation (sending traffic to better-performing variations), maximizing conversion lift during the testing period itself rather than waiting until a test concludes. ezbot is like a multi-armed bandit, but with much deeper contextual information -- as you'll see below, it's more than just that.
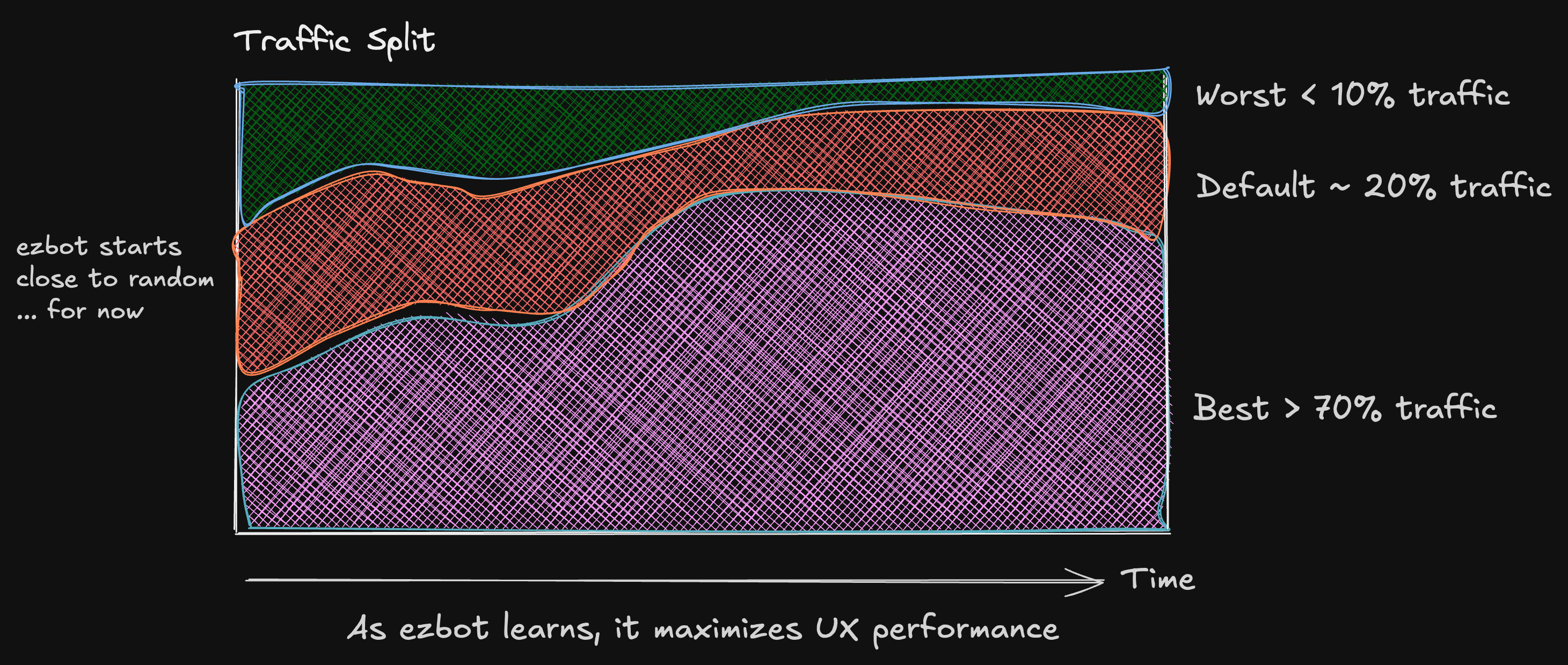
Personalized Experience Orchestration
Perhaps the most significant advancement is the shift from segment-based optimization to true 1:1 personalization. AI systems can determine which combination of elements will most likely resonate with each individual user based on their unique behavior patterns, contextual factors, and similarity to other users who have exhibited comparable behaviors. ezbot's unique approach personalizes the entire user experience in one shot.
Real-World Applications and Results
Organizations implementing AI-powered testing are seeing remarkable results across various industries:
E-commerce Product Discovery
A online retailer implemented ezbot's AI-powered system that dynamically adjusts butter-bar calls-to-actions, add-to-cart buttons, and newsletter popups based on individual user behavior. The system continuously tests different approaches for different user types, resulting in a 100% increase in add-to-carts in 30 days.
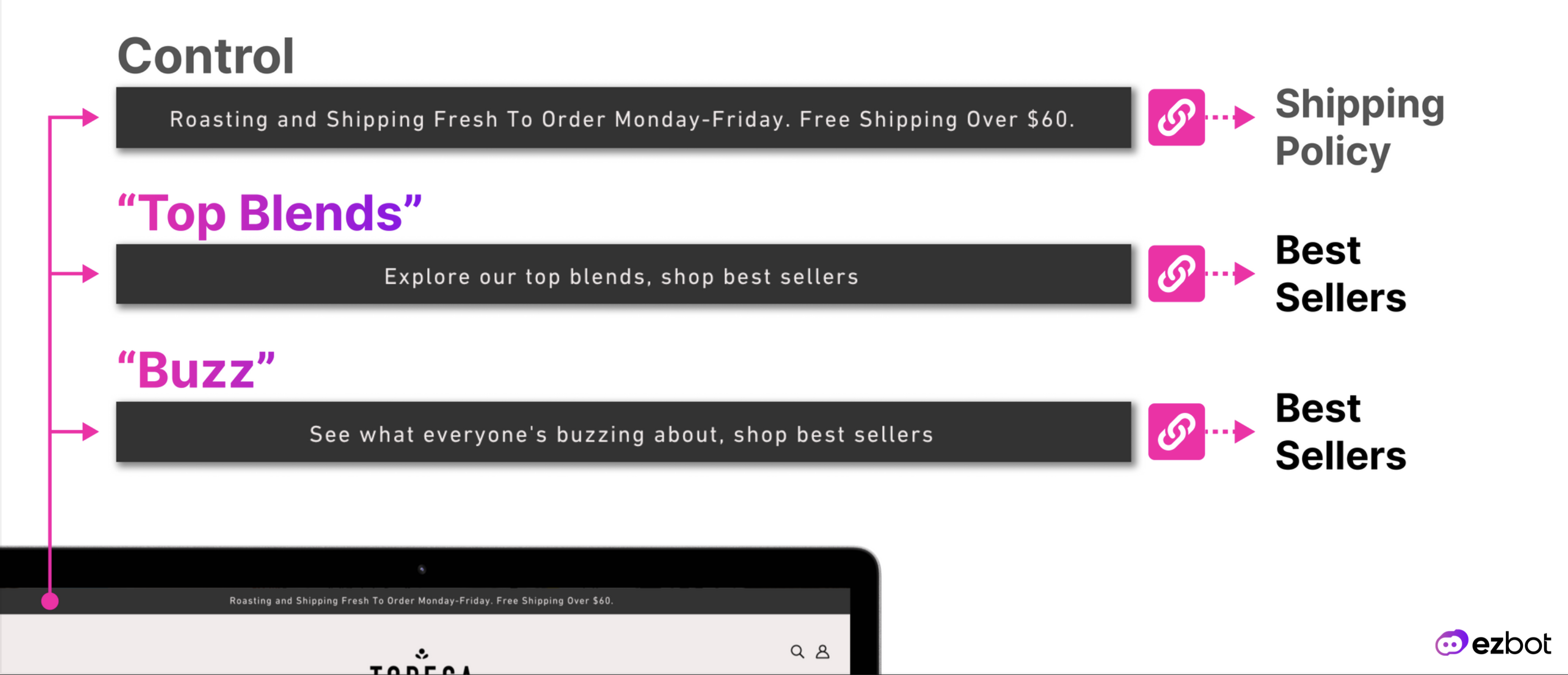
B2B Lead Generation Optimization
A SaaS company deployed ezbot's AI platform to optimize its lead generation funnel. The system automatically identified the optimal combination of headline, annual/monthly pricing, social proof elements, call-to-action design, and feature highlights for different visitors. By using traffic sources and inbound page paths, ezbot's system maintains a 10% lift every month over a static site, after already doubling the conversions on the baseline site through initial iterations.
Implementation Challenges and Solutions
Despite the compelling benefits, organizations often face several challenges when implementing AI-powered testing:
Challenge 1: Data Quality and Quantity Requirements
AI systems require substantial data to perform effectively. Organizations with lower traffic volumes or incomplete data collection may struggle to fully leverage these capabilities. The solution often involves starting with hybrid approaches that combine traditional testing methodologies with targeted AI applications in high-traffic areas, gradually expanding as data accumulates. ezbot simplifies this process; we establish clean, private data pipelines for each customer. Our pristine data lakes make for an ideal AI training environment.
Challenge 2: Technical Integration Complexity
Implementing AI-powered testing often requires deeper technical integration than traditional testing tools. Server-side implementation, data pipeline development, and integration with existing martech stacks add complexity to deployment. Forward-thinking organizations are addressing this by developing phased implementation roadmaps with clearly defined milestones, often starting with cloud-based solutions that minimize internal technical requirements. Again, ezbot makes this process easy. Our Javascript snippet works alongside any other martech tool, installing in minutes and taking control over whichever elements you choose on your site.
Challenge 3: Organizational Adaptation
Perhaps the most significant challenge is organizational. Teams accustomed to traditional testing methodologies must adapt to new workflows, develop new skills, and embrace a more automated approach to optimization. This requires investment in training, revised processes, and often cultural changes around data-driven decision-making.
The Future of AI-Powered Testing
Looking ahead, several emerging trends will likely shape the continued evolution of AI in digital experience optimization:
Cross-Platform Experience Orchestration
As AI systems mature, they will increasingly orchestrate consistent yet optimized experiences across websites, mobile apps, email, in-store digital displays, and other touchpoints. This omnichannel orchestration will ensure that insights gained in one channel can inform optimization in others, creating truly seamless customer journeys.
Ethical AI and Transparent Personalization
As personalization becomes more sophisticated, questions about transparency, consent, and potential manipulation are growing. Future AI testing platforms will likely place greater emphasis on "explainable AI" that can articulate the rationale behind personalization decisions and provide users with greater control over their personalized experiences.
Predictive Intent Optimization
Rather than simply optimizing based on past behaviors, next-generation AI testing will increasingly focus on predicting future intent—understanding not just what users have done but what they're likely trying to accomplish, even before they explicitly signal their goals. This predictive approach will enable truly anticipatory experiences that meet user needs before they're articulated.
Implementing AI-Powered Testing in Your Organization
For organizations looking to harness these capabilities, several steps can help ensure successful implementation:
1. Audit your data foundation. Assess the quality, completeness, and accessibility of your customer data before investing in AI-powered testing platforms (or find a platform like ezbot that does the hard work for you)
2. Start with high-impact, high-traffic areas. Focus initial implementation on areas with sufficient data volume and clear business impact potential.
3. Develop cross-functional expertise. Build teams that blend data science knowledge with traditional CRO expertise and business acumen.
4. Establish clear success metrics. Define how you'll measure the impact of AI-powered testing beyond traditional conversion metrics.
5. Create a roadmap for progressive implementation. Plan a phased approach that expands capabilities as your organization develops expertise and confirms value.
How ezbot is Shaping the Future of AI-Powered Testing
Among the companies pioneering this space, ezbot stands out for its innovative AI-powered testing and optimization approach. Unlike traditional tools that require extensive human configuration, ezbot uses advanced AI to autonomously identify optimal combinations of UX elements for each individual user. The platform leverages contextual data—including information about when, where, and how users enter the site—to deliver truly personalized experiences in real-time.
This contextual intelligence enables ezbot to go beyond simple personalization based on past behavior, incorporating situational awareness that considers immediate user circumstances. For organizations struggling with limited testing resources or data science expertise, ezbot offers an accessible entry point into sophisticated AI-powered optimization without requiring extensive technical implementation or specialized skills. If you're interested in learning more, please reach out.
Conclusion
AI-powered testing represents far more than an incremental improvement to existing optimization methodologies—it fundamentally transforms how organizations approach digital experience enhancement. By automating insight discovery, enabling true 1:1 personalization, and continuously optimizing experiences in real time, these technologies are setting new standards for conversion rate optimization.
Organizations that embrace these capabilities will gain significant competitive advantages through superior customer experiences, higher conversion rates, and more efficient optimization processes. Those who remain wedded to traditional testing methodologies risk falling behind as their competitors leverage AI to develop deeper customer insights and more compelling digital experiences.
The future of optimization is not just about testing more variations or achieving incremental improvements—it's about creating adaptive digital experiences that continuously evolve to meet each user's unique needs and preferences. ezbot and AI-powered testing is making that future a reality today.
👉 We wrote a blog series on this topic! Check out the first post: how conversion rate optimization is changing for non-technical teams.